12 Best Machine Learning Strategies for E-commerce Businesses
In today’s world of E-commerce, it’s impossible to run a successful online shop without leveraging Machine Learning.
Check out this article to find out how to incorporate artificial intelligence into your
e-Business in these easy ways:
- For price optimization
- For search result optimization
- For product recommendation
- And many more
Let’s get started!
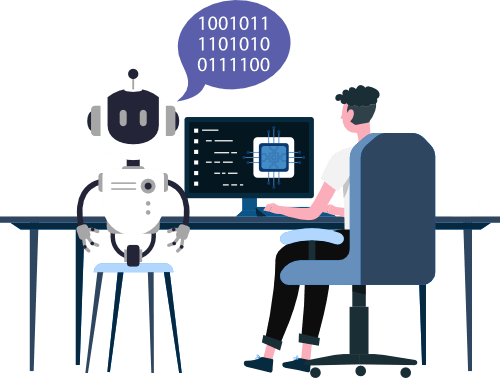
- How machine learning works – AI Use cases in E-commerce
- 1. Pricing Optimization
- 2. Segmenting, Personalization and Targeting Customers
- 3. Search Results Optimization
- 4. Product Recommendations
- 5. Predictions About Your Customers
- 6. Site Search Autocomplete
- 7. A/B tests using AI
- 8. Chatbots for Automated Customer Support
- 9. Inventory Management
- 10. Omnichannel Marketing Boosting with ML
- 11. Image Processing and Recognition
- 12. Fraud Protection
- Conclusion
Today, when global E-commerce sales amount to $4.88 trillion, it’s an innovator’s game.
According to Gartner, up to 80% of customer interactions are managed by AI today.
Introducing AI into sales, marketing, even inventory management is both a natural next step in the evolution of commerce and an absolute must for those who want to keep up and make cost-effective decisions.
And in this article, we are going to show you how you could effectively be leveraging AI in your E-commerce business.
Let’s kick off with the answer to….
How machine learning works –
AI Use cases in E-commerce
If we want to define machine learning in the simplest terms, it is the study and creation of algorithms that can learn from given sets of data and extrapolate from those to predict trends and outcomes.
This is a necessary function of any algorithm-based system that has to deal with large, frequently changing data sets – from natural language searches (where wording, typos, and new phrases are common) to facial recognition (where the system basically never meets the same input twice, but still has to determine the key features). Machine learning is used in email filtering, weather forecasts, diagnosing disease and predicting socioeconomic trends.
E-commerce is a field in dire need of machine learning. Even a small webshop can create millions of relevant data points daily that a single human, or even a team of experts could never hope to fully comprehend and utilize.
But, a machine can do it.
It will not only monitor these data sets, changes and trends in them, but make connections.
An AI based on machine learning will reason. Deduce, based on experience.
For example, it can help with…
1. Pricing Optimization
Price is, unsurprisingly, one of the most important factors when considering a purchase. In fact, price is one of the major drivers for at least 47% of customers according to BigCommerce.
If your prices are high – or just higher than your competitors’ – your cart abandonment rate can increase, and even more so if you don’t set shipping prices correctly. If your store offers international shipping, you also have to display prices based on the location of the customer.
There are many other factors that may influence your prices – supply and demand, which promotions are running, what time of the day or year it is and so on.
Via machine learning, these factors could be assessed in a fraction of a second and your site will display dynamic pricing: showing the customer the most up-to-date price for them based on the aforementioned factors.
This ensures prices are instantly understood (no “how much is that in my currency”, “does that include shipping” etc.), with no surprises, and no need for you to manually change them.
2. Segmenting, Personalization and Targeting Customers
In traditional brick-and-mortar stores, segmenting was done solely by salesmen, who approached the customers. By observing and asking a few questions, they could gather more than enough information about demographics, their needs and doubts, and their overall mood based on non-verbal communication.
This made it possible to immediately address any concerns about the products, matching the words and expressions used by the customer, being able to upsell them on the spot, and reinforcing their purchase intent.
When the customer has to deal with an online experience, all of this has to be replaced, and scaled.
Segmentation has to be based on behavior that can be measured.
And on-site search is a great place to start.
When searching, shoppers use natural language, which gives clues about their background and native language. Based on the results they click on, an algorithm can determine what they are looking for and offer more relevant results, which encourages them to make a purchase.
And based on what other customers in the store previously bought, in conjunction with this particular search, cross- and upsell offers could be made with great confidence. Part of the demographic information is readily available as soon as someone signs up – most times you will know their age, location and whatever data you ask for.
You can also monitor their behavior on your site – what content do they read, what materials do the download, how often do they return and how often do they make a purchase.
You can also keep track of the kind of emails they open and when are they most likely to make a purchase during a day, a week or year.
There is an incredible amount of data generated from all these interactions – and machine learning is what makes it possible to assess behaviors to ascertain patterns.
These patterns help you make sense of data sets and make more effective marketing decisions. For example, you can create ultra-targeted campaigns with the most relevant messages, and increase your conversion rate by offering customers exactly what they are looking for.
3. Search Results Optimization
Providing search results based on keywords is just the very first, most basic step in on-site search. In order to provide shoppers with the best experience possible, your search should go much deeper than that.
Given a large enough data set, you could determine which results are better for people in certain locations, how to optimize E-commerce search result filters, and which products best match their needs based on their previous behaviour.
By analyzing the data and figuring out what items go together, you can also recommend similar products, and even cross-sell items that are frequently bought together by your users.
Machine learning is able to pinpoint the trends and patterns needed to automatically determine these.
Leveraging this leads to higher click-through rates on your results pages, higher conversion rates, and a higher average order value.
It’s worth noting that filtering results in real-time based on user inputs, via faceted search, paired with AI-based recommendations is currently the single most effective method of providing relevant results.
But let’s stay for a minute with recommendations…
4. Product Recommendations
If you’re curious about how effective automatic product recommendations are, look no further than Netflix. According to McKinsey, 75% of what people watch on the streaming platform is suggested to them via an algorithm that analyzes user behavior. (The same is true for 35% of purchases at Amazon.)
You might think that determining which products go well together is easy – for example, simply track what people watch after they finish Futurama, and if it’s Rick and Morty, recommend that, and that’s it.
But you should also take into account the demographics – people in non-English speaking countries might go for movies and series in their native language with more enthusiasm. The preferences of young and older audiences will differ, as will the taste of those living in East Coast metropolitan areas versus those in rural European towns.
And this doesn’t even account for personal taste of genres, styles, lengths, eras etc.
To be able to offer ultra-targeted products to your customer, you have to work with a very large number of variables, each weighed differently.
It is virtually impossible to do this without a machine learning algorithm – even if you have just a few hundred products.
As you can see, knowing your customers is a bit like predicting the future.
To prove the importance of providing the shopper with the right product suggestions throughout the customer journey, check how significantly Rossmann’s conversion rate and average order value improved by utilizing AI-backed recommendations:
5. Predictions About Your Customers
Machine learning can tell you a lot of things about the people who visit your site and make a purchase – even things like how likely they are to buy from you again or what they might be interested in.
Check out what machine learning can predict.
Customer Lifetime Value Prediction
To fine-tune your communication and messages, it’s useful to know how much money a customer is likely to spend in your store over a given time period.
If you can estimate a lifetime value based on behavior, you can also make your marketing more cost-effective and targeted.
You can also identify customers who are the most valuable and deserve special attention.
Predicting if a customer will make a purchase
Imagine this: you have an online store for office supplies. You have a customer who orders the same amount of ink cartridges from you about every 6 months.
Let’s say 5 months pass, and this customer logs into your site, but doesn’t order anything. Logically, this could mean that they are checking the prices in order to budget the next order, or they might be comparing your prices to your competitors’.
As a store owner, you most likely will never learn that they’ve logged in and done this.
However, the AI will notice it.
And it can deduce that this is the right time to provide a little incentive to increase average order value – as the customer is a returning, but hesitant one, maybe it’s time to fire up a workflow that provides them with a personal discount for their next order, thanking them for their loyalty.
Predicting customer return (and purchases)
If, based on their behavior, a customer is likely to return to your store later, then an entirely different marketing message may resonate better with them.
Algorithms can predict, on the long term, whether this is the case and can initiate longer workflows with messages that target loyalty and reinforce branding.
Customer churn prediction
Retaining existing customers is one of the most important tasks if you want to keep your marketing budget from bleeding. Acquiring new customers is much most cost-intensive.
A machine learning algorithm can determine which shoppers are the most likely to abandon your site – based on things behaviour such as: returning to your store less frequently, smaller purchases, etc.
When the AI realizes this is the case, it can initiate workflows targeted at retention, giving shoppers incentives to stay.
Client size prediction
Based on average order value and purchase frequency, along with other information like number of employees, and company type, an algorithm can estimate the size of the client for you.
This gives you great insight into deciphering which potential customers to pay special attention to. You can make more personalized offers and give them deals that are more cost-efficient in the long term.
As you can see, machine learning really tells your future by predicting what your customers will or won’t do.
Another forward-looking application of machine learning that deserves its own subsection is…
6. Site Search Autocomplete
A truly useful autocomplete has to learn, instead of scouring through different product attributes and description.
It has to understand the natural language of the users instead of the often technical and machine-like phrases of database entries.
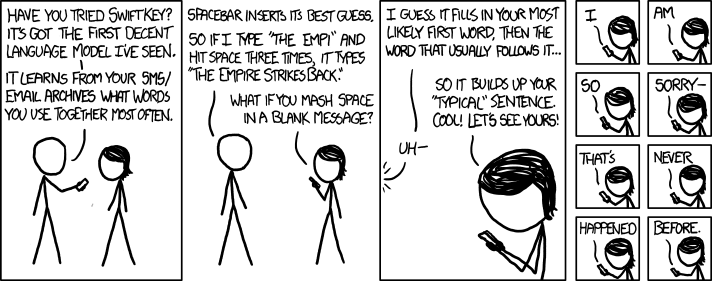
E-commerce search best practices dictate that online stores use an artificial intelligence-based autocomplete as it streamlines the shopping journey and provides shoppers with a sense of comfort as this is what they’ve come to expect.
So, natural language processing and machine learning have to understand the kind of language and phrases your customers use, how often they use them, if the results are satisfactory for those phrases, and even common typos along with the correct spelling.
This way, every user can feel that the search engine is truly there to help and pays attention to the smallest details, all without being annoying.
Speaking of details…
7. A/B tests using AI
A/B testing is a powerful tool in online marketing, but it can also be tricky.
Let’s say you want to run an A/B test for a product page.
Firstly: what do you change? The display of pricing? The location of your CTAs? The background color?
You see, if you change more than one thing, you can’t really be sure what causes the next positive or negative change.
But, if you change only one thing, the change may be so small it can’t even be registered.
And what KPIs should you track?
Yes, conversion and purchase rate are the most obvious numbers to track.
But time spent on page, number of clicks, and return rate are also important.
Machine learning and AI makes this testing process easier:
- Based on historical data, it decides which elements should be tested and automatically creates variants.
- It can dynamically change page elements based on test results. For example, displaying pages differently for different demographics or locations.
It can find the optimal versions much faster, because it can take all the variables into account and find the connections between even small changes.
8. Chatbots for Automated Customer Support
With customer support, there rarely is an optimal choice. If you want to solve all issues with manpower, your support team will be huge and expensive – and not at all efficient, as many times they will be dealing with things that could be solved by redirecting the customer to an FAQ page.
On the other hand, you can’t completely automate support, because a lot of issues will require human assistance, and your customers will quickly get annoyed if they can’t get it.
On the other hand, you can’t completely automate support, because a lot of issues will require human assistance, and your customers will quickly get annoyed if they can’t get it.
The solution to this problem is often implementing a chatbot based on machine learning.
These chatbots are able to maintain a conversation with the customer. Not only by using previously defined answers, but also via AI – they’re able to learn about natural language from every conversation.
Of course the chatbot will require time to learn, to get to know the products and services as well as the customers and their way of communication.
It will never pass a Turing test, but it could, in time, identify opportunities for upsell, create customized coupons, and open tickets for humans in customer support to handle.
Keep in mind, however…
A professional custom chatbot can cost anywhere between $30,000 and $300,000, depending on the desired functionality.
Speaking about money well spent…
Artificial Intelligence recently opened another avenue of sales for E-commerce stores by combining online shopping with chatbot assistance and messaging apps. This new means of shopping called conversational commerce, can not only enhance the customer experience, but improve conversion rates, and increase online sales overall. Check the video for more details:
9. Inventory Management
You know how a smart fridge reminds you you’re out of milk and puts it on your grocery list for you?
ML does that for E-commerce, times a billion.
Well, a trillion actually, as around 7% of the US GDP (more than $1.1 trillion) is tied up in cash in inventory along with accounts receivable and accounts payable.
Inventory management and logistics is nothing to sneeze at in terms of complexity.
If you run a successful online store, you have to monitor your stocks, reorder items, predict trends in demand, coordinate contractors, deal with manufacturers, suppliers, mailing services, and manage your revenues accordingly.
This is exactly the kind of job machine learning was made for.
By monitoring all your inventory, and even predicting future trends in supply, demand and even cash flow, you can be sure that with AI, you won’t be the airline that overbooks flights or flies empty.
You will be the airline that plans their next year schedule with absolute confidence.
10. Omnichannel Marketing Boosting with ML
Let’s get the obvious out of the way: omnichannel marketing brings you higher retention and conversion rates and boosts your revenue. But only if you use the available channels wisely.
Of course, you could just hire a marketing team, where there are dedicated people for social, email marketing, content creation, and tell everyone to give it 100%.
But is there a better way to do it?
Actually, yes.
Analyzing the data your customers create when interacting with your activities on those channels is majorly important.
Based on customer behavior – ads that perform well, content that’s frequently read, email open rates – machine learning algorithms can analyze your messages and display them in a way that ensures every customer gets the perfect one.
Online real estate became more important than television and radio partly because of how results can be tracked and campaigns can be optimized.
ML is the natural next step in this evolution.
11. Image Processing and Recognition
Image recognition can be a great tool for an online store with thousands of products in their inventory.
Ideally, it enables a customer to just upload a photo they snapped of a given product at home or at the store. The system then processes it on the store’s servers, and instantly displays a response with availability, current price, shipping info so the shopper knows where to buy it.
More often than not, this leads to a purchase as it’s the most convenient shopping method.
Beauty.com, for example, was able to increase sales by 15% after implementing their visual search feature.
Another possible use of image processing is to give ultra-targeted recommendations. For example, processing a photo of the customer and then applying clothes available in store, so they can see how different styles would look on them.
12. Fraud Protection
Fraud is inseparable from commerce as a whole, and E-commerce is especially vulnerable.
Online shopping offers huge opportunities for those who want to take advantage of automated systems, which is why it is important to have algorithms in place that can detect fraudulent activity.
Integrating a CAPTCHA is not enough. You also have to monitor behavior and look at how certain people use your site.
Integrating a CAPTCHA is not enough. You also have to monitor behavior and look at how certain people use your site.
With machine learning, you can identify repetitive patterns that don’t match with human behaviour; such as: filling in forms too quickly, opening dozens of pages for split seconds, entering multiple different sets of information rapidly at checkout, etc.
Conclusion
Introducing artificial intelligence in E-commerce processes is not something futuristic or even a highly complex task. There are applications and services readily available for most functions that have been developed by people over the past decade.
It’s not always cheap, but it’s worth the cost.
- It will help you understand your customers and audience better
- Increase your sales and average order value
- Cut unnecessary work
- Give you deep insight that no human ever could
You don’t have to hand over everything at once to the machine of course. Start small. Implement a smart site search solution, start using recommendations based on machine learning – and get to the rest when you are ready.
But get moving, because the analogue methods are phasing out fast.